Synposis:
We present a validated AI technique for quantification of whole aorta diameter from non-cardiac-gated MRI. The mean absolute error between AI-predicted and radiologist-reported diameter is less than 0.27 and 0.249 cm, while pearson correlation is 0.72 for the ascending (p-value=0.0002) and 0.74 for the descending aorta (p-values=0.0012). SVM classifiers indicated that a 3.15 cm (AUC=0.93) threshold is used to detect a descending aortic aneurysm while a 4.3 cm (AUC=0.92) threshold is used for ascending aortic aneurysm. Large-scale analysis showed aortic diameter to increase with age.
Summary:
Automated AI method to predict aortic diameter having good agreement with those measured by clinicians is proposed. SVM classifier is used to detect aortic aneurysms. The diameter of the aorta increases with age for both genders.
Introduction:
The aorta is the biggest artery in the body, and the pathologies such as aneurysms, stenosis and dissections can be life threatening due to the high pressure of blood flowing through its branches. Pathologies of the aorta if not detected early, may require surgical intervention often as an emergency procedure [1].
Quantification of the aorta in radiographic images such as MRI or CT scan is clinically relevant [2-3]. The conventional methods which include edge detection and mathematical techniques to detect the aortic centerline to measure the diameter, helps to reduce measurement time and variability. However, these methods are not fully-automatic, requiring user interaction and experience in medical imaging [4].
In this work, we develop and validate a fully automated artificial intelligence (AI)–based system to predict the diameters of the ascending and descending aorta having good agreement with the diameters reported by clinicians. The AI model was used to determine the best image slice on the non-cardiac-gated MRI to analyze, segment the regions, extract multiple diameter measurements along the entire length of the ascending and descending aorta, and output the largest diameter for each aortic segment. The predicted diameters were then used to classify the subjects according to the presence or absence of aneurysms on the basis of predetermined thresholds defined by SVM (Support-Vector-Machine) classifiers.
Methods:
To train, validate and test deep learning-based segmentation’s performance, a training subset of 134 axial torso T2-weighted non-gated MRI was utilized; these sequences were obtained as part of a Whole-Body MRI (WB-MRI) protocol within a preventative health screening program. To validate the performance of AI-based quantification of aortic measures, we applied solutions over our larger cohort consisting of normal heart subjects (3485; male=1788; female=1697) subjects. Among them, 48 subjects were reported with ascending and descending aortic aneurysms. MRI scans were performed on a 1.5 Tesla Siemens Espree MRI machine. No contrast agent was used. Each 3D scan consists of 144 axial slices with each slice having a voxel size of 1.67 x 1.67 x 5mm. Automatic quantifications which include aortic diameters and their normalization with Body Surface Area (BSA) were performed using segmentation masks of the whole aortic structure. Linear regression models were used to evaluate the relationship between aortic diameter and age.
Results:
We used Dice score as a metric for reporting performance of the trained 3D UNet-based model on unseen test images. We achieved up to 0.95, 0.91, and 0.89 Dice scores for ascending, arch and descending aorta including abdominal part, respectively. Fig.1 shows the entire aortic structure segmentation from axial T2 non-gated MRI with red, blue, and pink labels representing ascending, descending, arch and abdominal aorta. The visualization panels were (a) the 3D aortic structure, the overlay of segmentation into raw MRI in axial (b), in sagittal (c), and coronal (d).
After the segmentation mask was extracted, the follow-up measurements were performed to quantify the volumetric features that support radiologists in making clinical decisions. For each sub-structure of the aorta, we measured the cross-sectional areas and its corresponding diameter. In addition, we reported the maximum cross-sectional diameter as well as the plain where the maximum diameter was captured to support the radiologist. The Pearson correlation between AI-based and radiologist-based measures were equal at 0.72 (p-values=0.0002) for the ascending aorta and greater than 0.74 (p-values=0.0012) for the descending aorta. Fig. 2 shows Bland-Altman plots of the difference between the reported and predicted diameters for ascending aorta (left) and (right) descending aorta.
Large-scale analysis findings presented in Fig. 3 have revealed the absolute (upper) and normalized (lower) descending aorta diameter gradually increases with age for both genders with a larger diameter (about 0.2-0.4 cm) in males compared to females. Fig. 3 also analyzed the changes in absolute descending diameters (cm) per decade from 20 to 80 years as 2.45, 2.59, 2.76, 2.93, to 3.1, and 3.21 cm for males and 2.22, 2.30, 2.43, 2.60, 2.74, and 2.84 cm for females. The regression analysis reveals that the descending aorta diameter increases about 4-10% every decade as shown in Fig. 3.
We performed classification of ascending and descending aorta aneurysm and ascending and descending aorta in normal subjects using SVM methods and aortic diameters as features. By using the boundary decisions defined by SVM classifiers in Fig. 4, the threshold for ascending and descending aorta aneurysms are 4.3 cm and 3.15 cm, respectively. On average, obtained AUCs presented in Fig. 5 are 0.92 and 0.93 for ascending aorta and descending aorta aneurysm classification, respectively.
Conclusion:
To our knowledge, this is the first work to tackle the challenges in automatic quantification of aorta and aortic aneurysm detection using large-scale non-cardiac-gated MRI dataset. Our proposed framework for automatic quantification of aorta and detection of aortic aneurysm may provide support to radiologists by improving quantitative reproducibility and read-time efficiency for aortic aneurysm detection from screening non-cardiac gated MRI. Our future investigation could include the effect of BSA on the vascular diameter.
Acknowledgment:
We would like to thank the MRI Technologists, Patient Care, and Backend teams for their contributions in data acquisition.
References:
[1] Bezerra de Carvalho Macruz, Fabiola, et al. "Quantification of the Thoracic Aorta and Detection of Aneurysm at CT: Development and Validation of a Fully Automatic Methodology." Radiology: Artificial Intelligence 4.2 (2022): e210076.
[2] Turkbey, Evrim B., et al. "Determinants and normal values of ascending aortic diameter by age, gender, and race/ethnicity in the Multi‐Ethnic Study of Atherosclerosis (MESA)." Journal of Magnetic Resonance Imaging 39.2 (2014): 360-368.
[3] Rueckel, J., et al. "Artificial intelligence assistance improves reporting efficiency of thoracic aortic aneurysm CT follow-up." European Journal of Radiology 134 (2021): 109424.
[4] Raffort, Juliette, et al. "Artificial intelligence in abdominal aortic aneurysm." Journal of vascular surgery 72.1 (2020): 321-333.].
[5] Hahn, Lewis D., Kathrin Baeumler, and Albert Hsiao. "Artificial intelligence and machine learning in aortic disease." Current Opinion in Cardiology 36.6 (2021): 695-703.
Tables and Figures:
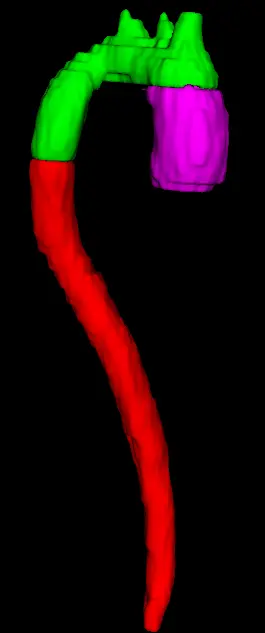
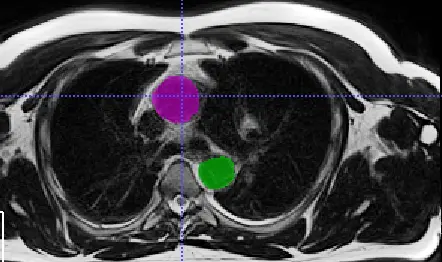
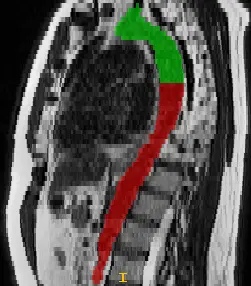
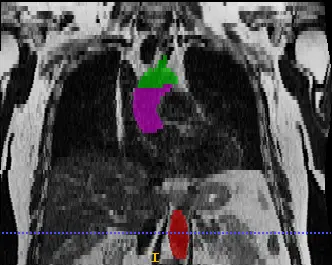
Fig. 1 The aortic structure segmentation from axial T2 non-gated MRI; red: descending and abdominal aorta, blue: arch of aorta, pink: ascending aorta. (a) the 3D aortic structure. (b) the overlay of segmentation into raw MRI in axial (b), in sagittal (c), and coronal (d).
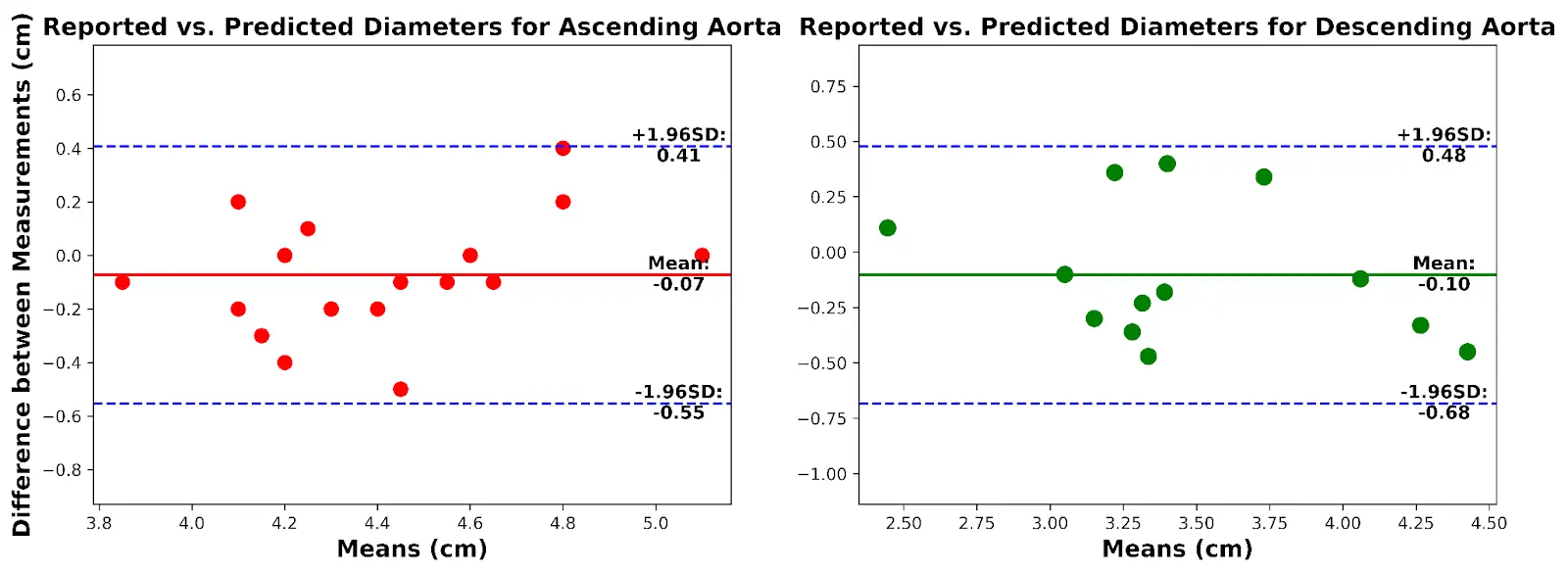
Fig 2. Bland-Altman plots of the difference between the reported and predicted diameters for ascending aorta (left) and (right) descending aorta. The x-axis represents the mean of the two measurements (cm), and the y-axis represents the difference (cm) between the two measurements. The red and green line represents the mean value of the differences, and the blue dashed lines represent the limits of agreement (±1.96 standard deviation of the mean difference).
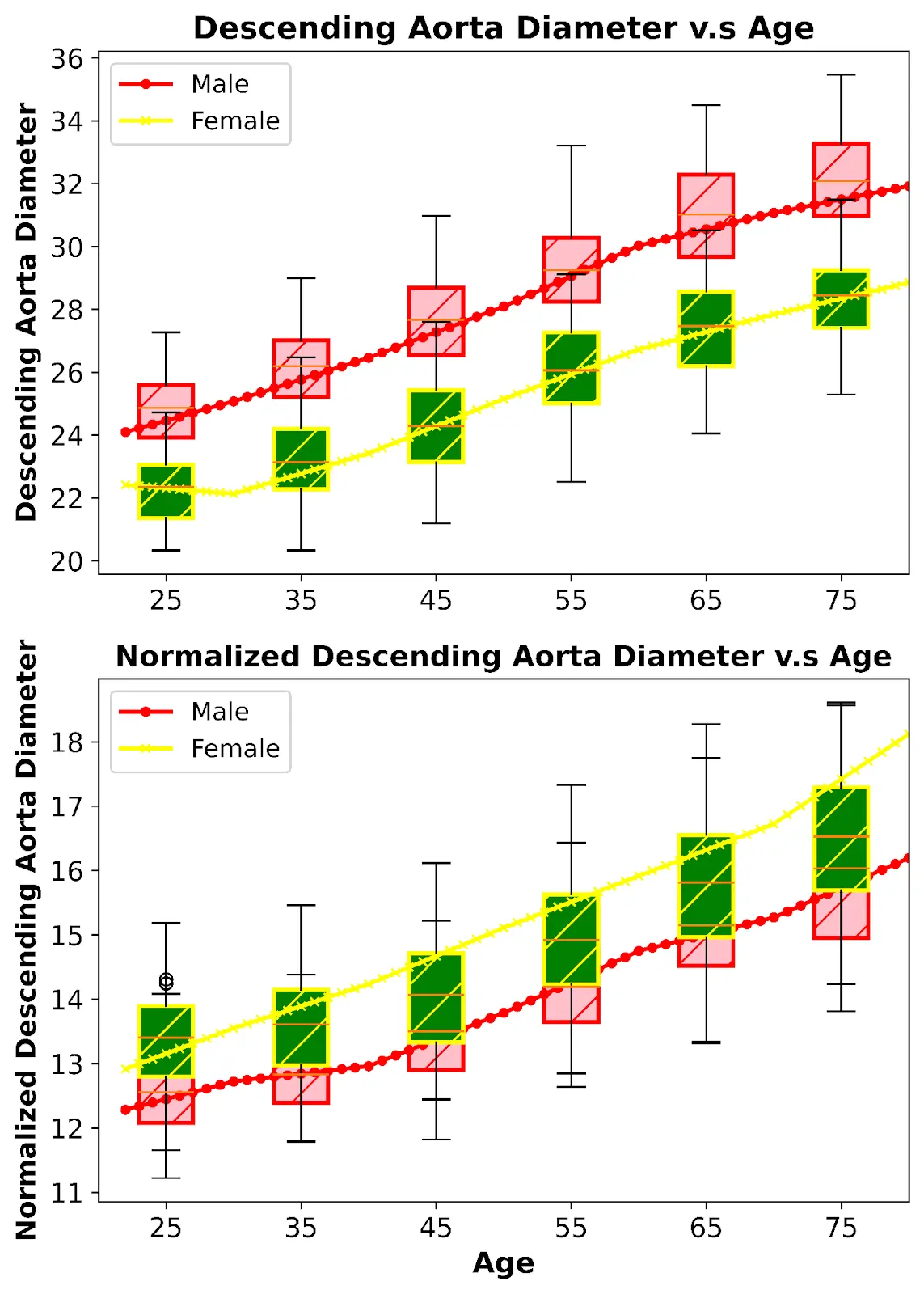
Fig. 3 The large-scale analysis of 3485 normal subjects on absolute descending aorta diameter and its normalization with BSA shows the increase of aorta diameters with age between males and females.
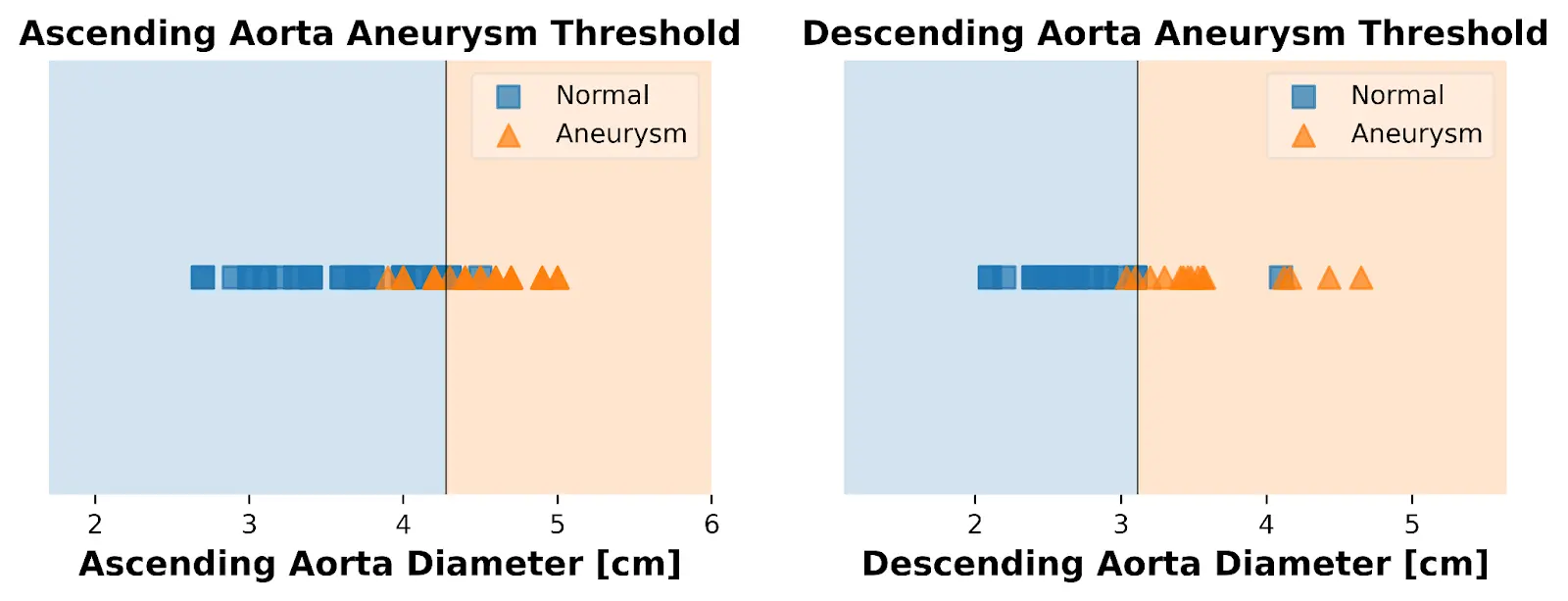
Fig 4. The boundary decision plots of SVM classifiers define thresholds for ascending and descending aortic aneurysm. As the plots show, the threshold for ascending and descending aorta aneurysms are 4.4 cm and 3.15 cm, respectively.
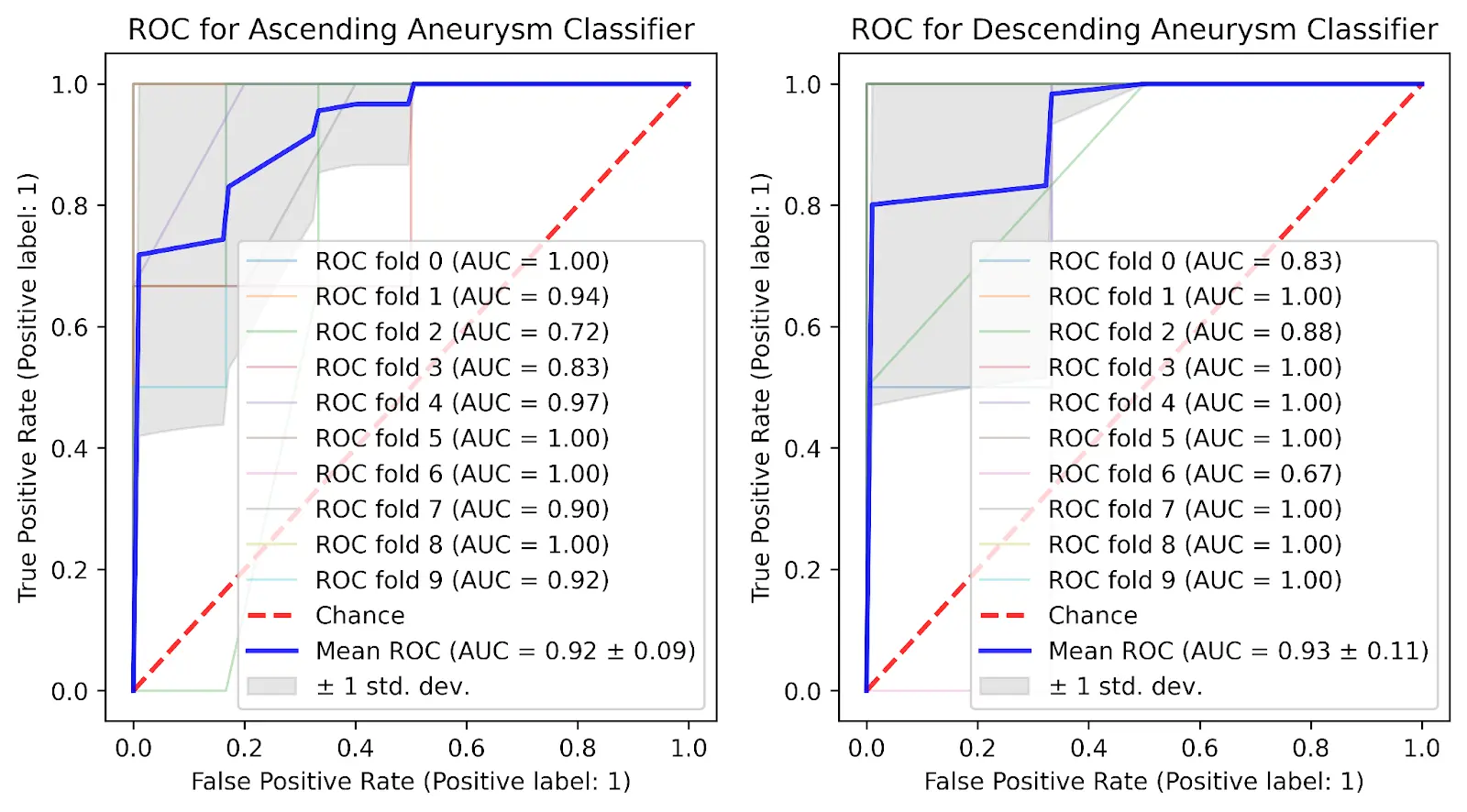
Fig 5. ROC plots using SVM classifiers for ascending aorta (left) and descending aorta (right) aneurysm classification. On average, AUC obtained are 0.92 and 0.93 for ascending and descending aorta aneurysm classification, respectively.