Synopsis:
Despite its ubiquity, cardiac assessment of non-cardiac-gated MRI has yet to be standardized, leading to undervaluation of the utility of whole organ assessment. This paper examines the feasibility of AI for automatic 3D volumetric cardiac quantification used to detect and stratify cardiomegaly on non-cardiac-gated MRI. Using AI, we automatically measure the cardiothoracic ratio and 3D cardiac volumetric features as indicators for detection of cardiomegaly. Large-scale results on 3485 healthy individuals revealed the effect of aging on cardiac features in both men and women. AI findings on non-cardiac-gated imaging offers useful and quantifiable information, increasing the diagnostic precision, and allowing its use for diagnosing cardiomegaly.
Summary:
Automated 3D cardiac volumetric quantification with non-cardiac-gated MRI using AI were introduced. Thresholds for detection and stratification of cardiomegaly conditions based on the extracted cardiothoracic ratio and absolute heart diameter were recommended.
Introduction:
A variety of conditions from congestive heart failure to congenital heart disease can lead to cardiomegaly, which if detected and treated early can limit the morbidity and mortality and might reduce the need for surgery [1]. Despite its shortcomings as a simple gross estimate of overall cardiac size, assessment of cardiothoracic ratio (CTR) is still commonly utilized by radiologists to diagnose cardiomegaly [2-3]. Visual inspection alone for stratification of cardiomegaly is challenging, particularly when mild [4]. Although the method continues to be recommended for cardiac enlargement assessment, it is time-consuming and subjective [5]. As the heart is a 3D object, 1D measurement alone such as CTR can be misleading when there is multidirectional enlargement. This work introduces an automated AI-based pipeline to quantify 3D cardiac volumetric features and detect the presence of cardiomegaly from non-cardiac-gated MRI. We then propose a strategy to detect and stratify cardiomegaly alongwith prediction uncertainty of detection. In addition, we perform a large-scale analysis on healthy individuals to examine the age-related effects on cardiac volume.
Methods:
A dataset of 134 axial T2-weighted torso MRIs was used to train, validate and test performances of deep learning-based segmentation. To validate the performance of AI-based stratification of enlarged heart conditions, an additional dataset consisting of normal heart population (3485; male=1788; female=1697) and enlarged-heart population (228; male=150; female=78) are included. Among the 228 enlarged heart subjects, there were 9 subjects with moderate enlargement of the heart, the rest were classified as mild enlargement. MRI scans of the torso were acquired from a 1.5 Tesla Siemens Espree MRI machine. Each scan consists of 144 axial slices with each slice having a voxel size of 1.67 x 1.67 x 5mm. Automatic quantifications which include entire heart volume (HV), horizontal maximum heart diameter (HD) and thoracic diameter were performed using segmentation masks of the heart. The adjacent great vessels of the aorta and inferior vena cava were identified and excluded. In addition, linear regression was performed to evaluate the relationship between these cardiac volumetric features and age.
RESULTS:
The Dice scores of AI-based methods for cardiac segmentation are as follows: descending and abdominal aorta: 0.92, arch of aorta: 0.83, heart: 0.80, inferior vena-cava: 0.83, and superior vena-cava: 0.77. Using extracted 3D segmentations, automatic cardiac quantification can be performed. Table I presents results of cardiac quantification, showing larger quantitations of cardiac features in moderately-enlarged heart subjects as compared to mildly and healthy-heart populations. Fig. 1 and Fig. 2 visualizes the scattering distributions as well as the means and standard deviations of the CTR, HD, HV in our dataset. Interestingly, the results indicate that the CTR has a low correlation with heart measurements (diameter and volume). Correlations between CTR with heart volume, ventricle diameter, atrium diameter, and maximum heart diameter, and height are 0.24, 0.17, 0.02, 0.33, and 0.19, respectively. Further, CTR showed a poor correlation with demographic features such as age (0.07), weight (−0.06), gender (−0.01), and height (0.05). The large-scale analysis using 3485 subjects has revealed the effect of normal aging on cardiac features. Fig. 3 shows the boxplots of HD and HV for each decade while the corrected values of these features resulting from linear regression at each age are presented (red line for males and yellow for females). Our findings show that the maximum horizontal heart diameter and heart volume increases with age for both males and females. Particularly, the mean HD (mm) for each decade ranging from 20 to 80 years are 137.96, 143.94, 147.49, 149.74, 150.7, 151.03 for males while these changes in females are 124.24, 124.00, 127.14, 131,20, 132.6, 134.01. However, the slopes for these changes are different between genders and decades.Based on our analysis, the CTR threshold for moderate and mild cardiomegaly conditions are 0.55 and 0.5 respectively. Any patients who have a CTR greater or equal than 0.55 will tend to be diagnosed by the reporting radiologists as having a moderately enlarged heart while a healthy heart patient will have CTR below 0.5. Any CTR between 0.5 and 0.55 will be considered as mild enlarged heart.
Conclusion:
In this work, we introduced an end-to-end deep learning framework that is capable of automatically segmenting the multi-label cardiac structures. To the best of our knowledge, this is the first paper that tackles the challenges in automatic quantification and stratification of cardiac conditions using non-cardiac-gated MRI. The value of this study lies in enabling automated opportunistic characterization and tracking of cardiomegaly, in addition to the expected benefits of more standardized and precise radiologist reporting of cardiomegaly.While the technique developed in this study may offer diagnostic utility for characterization of cardiomegaly on non-dedicated cardiac MRI, the following limitations are noted. The ground truth utilized may have inconsistencies and inaccuracies. The shortage of the enlarged-heart sample and the use of CTR as the indicator for stratification of enlarged-heart conditions. Next steps in developing this automated assessment of cardiomegaly will seek to apply these advancements more granularly on a chamber-by-chamber assessment for more specificity.
Acknowledgment:
We would like to thank the MRI Technologists, Patient Care, and Backend teams for their contributions in data acquisition.
References:
[1] Pairash Saiviroonporn, Kanchanaporn Rodbangyang, Trongtum Tongdee, Warasinee Chaisangmongkon, Pakorn Yodprom, Thanogchai Siriapisith, Suwimon Wonglaksanapimon, and Phakphoom Thiravit. Cardiothoracic Ratio Measurement Using Artificial Intelligence: Observer and Method Validation Studies. BMC Medical Imaging, 21(1):1–11, 2021.[2] You Sung Kim, Hyun Jin Park, Seog Hee Park, Ho Jong Chun, and Byung Gil Choi. A CT Criteria of Cardiomegaly. Journal of the Korean Radiological Society, 57(3):235–238, 2007.[3] Paulius Simkus, Manuel Gutierrez Gimeno, Audra Banisauskaite, Jurate Noreikaite, David McCreavy, Diana Penha, and Monika Arzanauskaite. Limitations of Cardiothoracic Ratio Derived from Chest Radiographs to Predict Real Heart Size: Comparison with Magnetic Resonance Imaging. Insights into Imaging, 12(1):1–10, 2021.[4] Hota, Partha, and Scott Simpson. "Going beyond cardiomegaly: evaluation of cardiac chamber enlargement at non–electrocardiographically gated multidetector CT: current techniques, limitations, and clinical implications." Radiology: Cardiothoracic Imaging 1.1 (2019).[5] Soham S Sarpotdar. Cardiomegaly detection using deep convolutional neural networks with u-net. arXiv preprint arXiv:2205.11515, 2022.[6] Inoue K, Yoshii K, Ito H. Effect of aging on cardiothoracic ratio in women: a longitudinal study. Gerontology. 1999 Jan-Feb;45(1):53-8. doi: 10.1159/000022056. PMID: 9852382.[7] Potter JF, Elahi D, Tobin JD, Andres R. Effect of aging on the cardiothoracic ratio of men. J Am Geriatr Soc. 1982 Jun;30(6):404-9. doi: 10.1111/j.1532-5415.1982.tb02841.x. PMID: 7077024.
Tables and Figures:

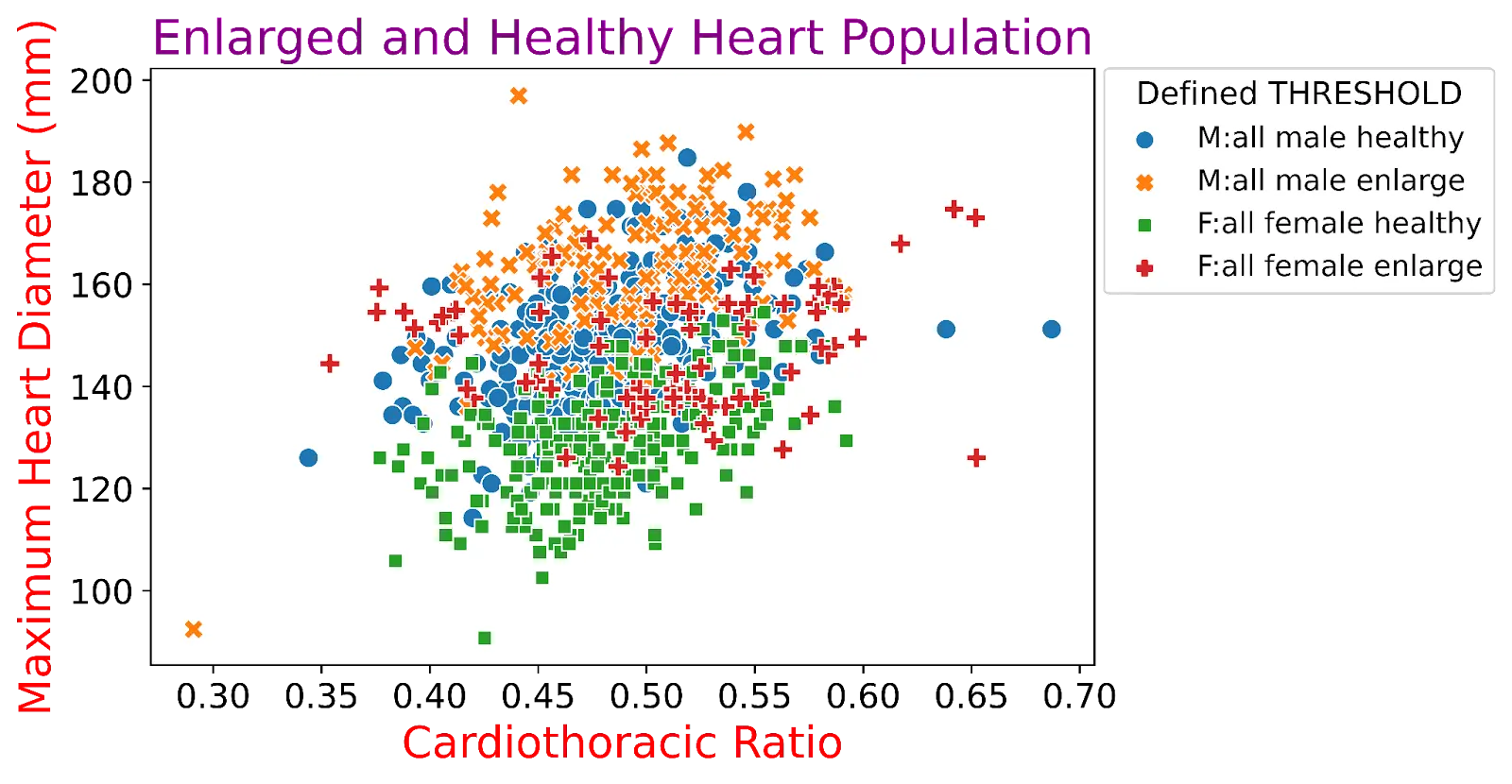


